
ABOUT ME
I am a research scientist working with Professors Elizabeth Barnes and James Hurrell at Colorado State University (CSU).
I currently work on eXplainable Artificial Intelligence (XAI) and its application to climate science.
In general, my research aims to improve understanding of how climate variability and change impact hydroclimate across scales and to assess societal risks that are associated with climatic hazards to inform adaptation and mitigation policies. My ultimate goal is to develop and use data science tools for reliable prediction and physical attribution of changes in hydroclimate and climatic extremes, towards mitigating and adapting to global environmental change. In the last decades, much progress has been made in this field, mainly stemming from more accurately and systematically monitoring Earth’s climate across scales and improving Earth System Models due to increases of computing power and physical understanding. Capitalizing on these advances, my research utilizes state of the art observations from space and land, reanalysis datasets and outputs from climate models of different complexity and uses a diverse set of approaches, including statistical and Bayesian analysis, machine/deep learning, and dynamical systems theory to pose and test hypotheses and improve predictive skill and risk assessment.
​
Specific research topics I have worked on include:
​
-
Data-driven exploration of sources of predictability for regional hydroclimate to improve prediction and reduce risk
My goal is to systematically identify sources of predictability of regional hydroclimatic variability to enhance physical understanding and improve S2S prediction. Other research questions include: How has/will climate change impact regional predictability by influencing climate modes and strength of teleconnections? What will be the effect of such changes of predictability on managing water resources and hydroclimatic risk? How to incorporate such non stationarities and possibly evolving dynamics into predictive data-driven algorithms (e.g., deep learning)? Can methods of explainable artificial intelligence be used to improve predictive skill and provide causality insights for hydroclimatic variability on sub-seasonal to seasonal timescales?
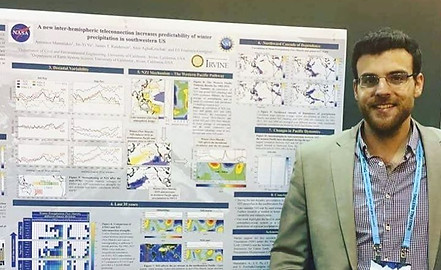
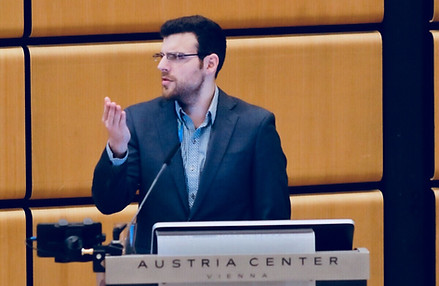

2. Long-term impacts of climate warming to global precipitation patterns
​
My goal is to increase our predictive understanding and physically-attribute past and ongoing decadal frequency-magnitude changes in the global distribution of precipitation under the effect of anthropogenic forcing and/or natural multi-decadal variability. I am specifically interested in past and future shifts of the Intertropical Convergence Zone (ITCZ) and the subtropical dry zones, and changes in the statistics of extremes. Research questions also include: Which of the past hydroclimatic changes can be attributed to multi-decadal natural variability and which to external forcing? How do systematic biases of climate models, e.g., the double-ITCZ, affect our assessment about future climatic change and especially precipitation? How can we identify and account for these biases in climate change attribution? How will future precipitation changes under different socioeconomic pathways or geoengineering scenarios impact the likelihood of extreme events like floods, droughts, wild fires, heatwaves, and their likelihood to co-occur (i.e., compound events)?
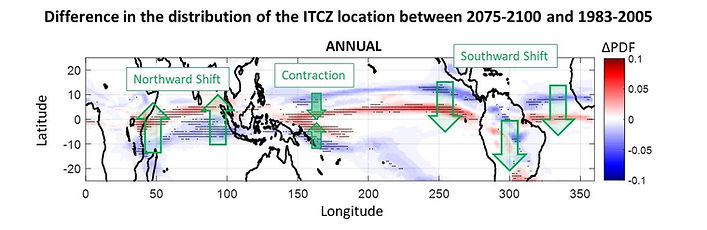
3. Pioneering the assessment and application of explainable Artificial Intelligence (xAI) methodologies to problems of hydroclimate
​
As it has been shown in the recent literature, neural networks have attracted great attention in climate science because of their ability to capture nonlinear system behavior and extract predictive spatiotemporal patterns. Given their black-box nature, however, and the need of scientists to physically interpret predictions, methods of explainable artificial intelligence are gaining popularity as a means to explain the network decision-making strategy.
​
​
​
​
​
​
​
​
​
​
​
​
​
​
​
​
​
​
​
I envision that attribution benchmarks can be used towards establishing best practices for XAI implementation. Future research questions regarding XAI include: How to quantify uncertainty of the network explanation in a best suited way for hydroclimatic applications? Can we use XAI methods to test the fidelity of knowledge guided AI? Can we use XAI techniques to infer causality in hydroclimatic applications?

In my recent research, I have developed an innovative framework to objectively assess the fidelity with which different XAI methods explain the network, an aspect that has been greatly overlooked before. Specifically, I proposed a generic approach to develop simple attribution benchmark datasets for benchmarking XAI methods. Attribution benchmark datasets consist of synthetic inputs and outputs, where the functional relationship between the two is known. This allows for deriving the ground truth (or at least a prior) of what the explanation of the network should look like for each prediction. In this way, the assessment of XAI methods is not based on subjective criteria, but rather it is based on the direct comparison of the XAI results with the ground truth of the explanation.
RESEARCH INTERESTS
EDUCATION
Precipitation predictability on S2S timescales
Sep 2016 - Sep 2020
University of California, Irvine, USA
PhD in Civil and Environmental Engineering
Future precipitation trends and shifts of the Inter-Tropical Convergence Zone (ITCZ)
Sep 2014 - Feb 2016
University of Patras, Greece
MSc in Water Resources and Environmental Engineering
Sep 2008 - Feb 2014
University of Patras, Greece
Diploma in Civil Engineering
Modeling of precipitation extremes
Bias correction of climate models outputs
Explainable Artificial Intelligence and its applications to climate science